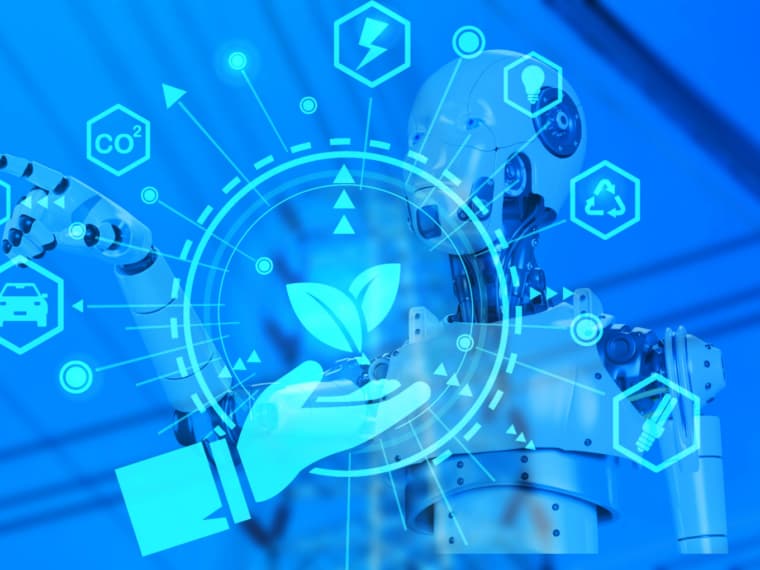
The climate economics index stress-tests reveal that climate change will impact 48 nations, encompassing 90% of the global economy, and evaluate their overall climate resilience
Machine learning (ML) and predictive analytics emerge as game-changers, offering a powerful arsenal for businesses to significantly reduce their environmental impact
The power of ML extends beyond monitoring. Predictive analytics can forecast future emissions based on historical data and current trends
The clock is ticking on climate change. Businesses face immense pressure to reduce their carbon footprint, but traditional methods often lack precision and struggle to keep pace with complex operations.
The climate economics index stress-tests reveal that climate change will impact 48 nations, encompassing 90% of the global economy, and evaluate their overall climate resilience. Major economic powers could face approximately a 10% reduction in GDP over the next three decades. If the temperature rises by 3.2°C, China is likely to experience a 24% decline in GDP by the middle of the century.
Likewise, the United States, Canada, and the United Kingdom are expected to suffer approximately a 10% loss each. Europe as a whole would undergo a slightly higher impact at 11%, with specific economies like Finland or Switzerland being 6% more vulnerable compared to France or Greece, which are at 13%.
Machine Learning And Predictive Analytics Emerge As Game Changers
Here’s where machine learning (ML) and predictive analytics emerge as game-changers, offering a powerful arsenal for businesses to significantly reduce their environmental impact.
Machine learning, a branch of artificial intelligence, allows computers to learn from data without explicit programming. Predictive analytics, leveraging ML algorithms, analyses past data to forecast future trends.
In the fight against climate change, this translates to pinpointing emission hotspots, predicting future carbon footprints, and optimising operations for maximum sustainability.
Traditional methods might struggle to pinpoint the exact source of energy waste in manufacturing units. ML algorithms, however, can analyse vast amounts of sensor data, identifying machines with abnormal energy consumption.
This empowers businesses to target specific areas for improvement, leading to significant reductions in energy use and carbon emissions.
The power of ML extends beyond monitoring. Predictive analytics can forecast future emissions based on historical data and current trends. This foresight allows businesses to proactively adjust operations, invest in renewable energy sources, or optimise transportation routes to minimise their environmental impact before emissions even occur.
Real-world applications abound. Buildings equipped with ML-powered systems can automatically adjust heating and cooling based on real-time occupancy and weather data, slashing energy consumption.
Key Advantages Of Using AI/ ML
According to the studies, building automation powered by AI can achieve energy savings of 10% to 30%. Logistics companies can leverage ML to design the most efficient delivery routes, reducing fuel use and emissions across their supply chain.
The research found that optimising logistics routes with ML can decrease transportation emissions by up to 20%. Businesses can even utilise ML to identify sustainable sourcing options, ensuring their entire operation minimises environmental impact.
The benefits are undeniable. By optimising operations, ML-powered solutions can lead to significant reductions in carbon footprint, often exceeding 20%. Reduced energy consumption translates to lower operational costs, boosting a company’s bottom line.
A study found that companies embracing AI for sustainability initiatives can achieve cost savings of 5% to 10%. Moreover, embracing sustainable practices enhances a company’s reputation and gives it a competitive edge in a market increasingly focused on environmental responsibility.
Conclusion
Data quality, access, and integration are crucial hurdles for the ML, Additionally, potential biases within ML algorithms necessitate careful design and implementation. However, these challenges are actively being addressed by climate tech companies and the continuous advancements in ML technology promise an even greater impact in the future.
The integration of ML with the Internet of Things (IoT) holds immense potential. Imagine a network of sensors constantly monitoring energy use in real time, feeding data into ML algorithms that can make instant adjustments for optimal efficiency. This future holds the promise of a seamlessly sustainable business landscape.
ML is a powerful tool that can revolutionise carbon reduction strategies. By embracing data-driven approaches and investing in ML solutions, businesses can significantly reduce their environmental footprint and pave the way for a more sustainable future. The time to act is now, and machine learning offers the key to unlocking a cleaner, greener tomorrow.